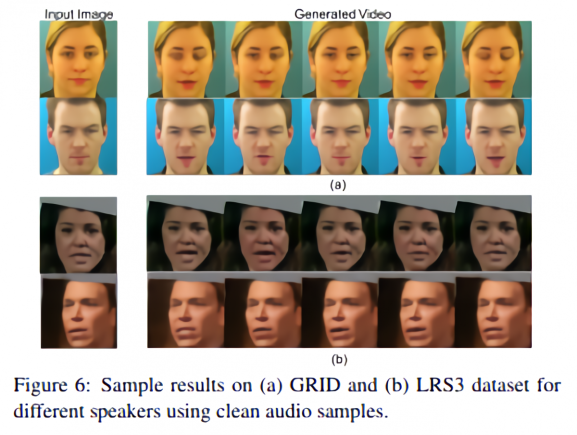
A growing body of research suggests that the facial movements of almost anyone can be synced to audio clips of speech, given a sufficiently large corpus. In June, applied scientists at Samsung detailed an end-to-end model capable of animating the eyebrows, mouth, and eyelashes, and cheeks in a person’s headshot. Only a few weeks later, Udacity revealed a system that automatically generates standup lecture videos from audio narration. And two years ago, Carnegie Mellon researchers published a paper describing an approach for transferring the facial movements from one person to another.
Building on this and other work, a Microsoft Research team this week laid out a technique they claim improves the fidelity of audio-driven talking heads animations. Previous head generation approaches required clean and relatively noise-free audio with a neutral tone, but the researchers say their method — which disentangles audio sequences into factors like phonetic content and background noise — can generalize to noisy and “emotionally rich” data samples.
“As we all know, speech is riddled with variations. Different people utter the same word in different contexts with varying duration, amplitude, tone and so on. In addition to linguistic (phonetic) content, speech carries abundant information revealing about the speaker’s emotional state, identity (gender, age, ethnicity) and personality to name a few,” explained the coauthors. “To the best of our knowledge, [ours] is the first approach of improving the performance from audio representation learning perspective.”
Underlying their proposed technique is a variational autoencoder (VAE) that learns latent representations. Input audio sequences are factorized by the VAE into different representations that encode content, emotion, and other factors of variations. Based on the input audio, a sequence of content representations are sampled from the distribution, which along with input face images are fed to a video generator to animate the face.
The researchers sourced three data sets to train and test the VAE: GRID, an audiovisual corpus containing 1,000 recordings each from 34 talkers; CREMA-D, which consists of 7,442 clips from 91 ethnically diverse actors; and LRS3, a database of over 100,000 spoken sentences from TED videos. They fed GRID and CREMA-D to the model to teach it disentangled phonetic and emotional representations, and then they evaluated the quality of generated videos using a pair of quantitative metrics, peak signal-to-noise ratio (PSNR) and Structural Similarity Index (SSIM).
The team says that their approach is on par, in terms of performance, on all metrics with other methods for clean, neutral spoken utterances. Moreover, they note that it’s able to perform consistently over the entire emotional spectrum, and that it’s compatible with all current state-of-the-art approaches for talking head generation.
“Our approach to variation-specific learnable priors is extensible to other speech factors such as identity and gender which can be explored as part of future work,” wrote the coauthors. “We validate our model by testing on noisy and emotional audio samples, and show that our approach significantly outperforms the current state-of-the-art in the presence of such audio variations.”