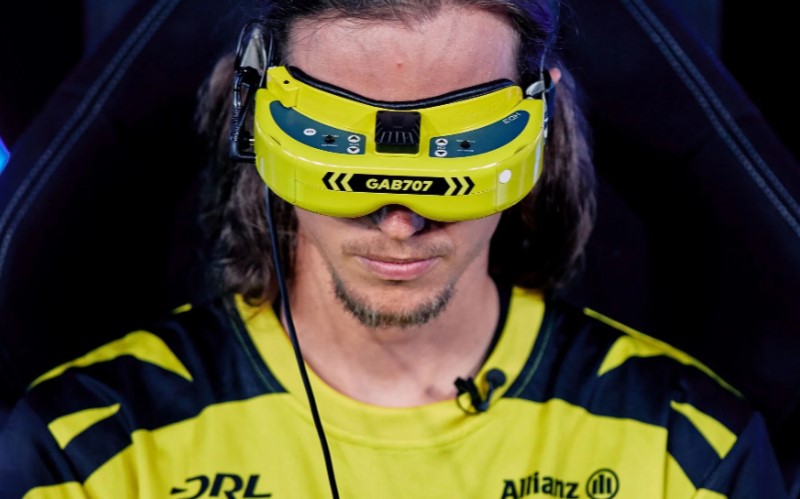
On Friday night in an old newspaper printing plant in Austin, the future of drone automation lifted off, accelerated and flew, nearly fast enough to beat one of the best drone pilots in the world.
Gabriel Kocher, known in the professional Drone Racing League as Gab707, sat behind a net, wearing video goggles and steering his drone through five square gates on a short, curvy course. Next to him were four teammates from the MavLAB of the Delft University of Technology in the Netherlands. They had already programmed their automated drone, which resembled a mini Stealth Bomber. Now they were watching to see if their code had made the drone fast and accurate enough to defeat Kocher.
It was human vs. machine and, for now at least, the human triumphed. Kocher guided his drone through the course in six seconds. It took MavLAB 11 seconds. Despite losing to Kocher, the four members of MAVLab—Federico Paredes Vallés, Guido de Croon, Christophe De Wagter, and Nilay Sheth—outdid five other teams that qualified for the AIRR (Artificial Intelligence Robotic Racing circuit) Championship and pocketed a $1 million prize from sponsor Lockheed Martin.
Afterward, Kocher was relieved to have won but conceded man’s superiority over the machine will not last forever. It’s a matter of time, experts say, before automated drones will whir past humans in increasingly popular competitions hosted by the Drone Racing League—and before they can use similar technology to handle complicated tasks in real life.
The latter possibility led Lockheed Martin to sponsor the AIRR circuit for coders, professors, students, physicists, and pilots to power automated drones. Remotely piloted Lockheed Martin drones drop missiles, help police and firefighters, and assist in rescue missions for missing persons. Lockheed Martin’s Keith Lynn, manager of the automated drone challenge, says automated drones will be better equipped to handle complicated rescue missions in areas where transportation routes and communication lines have broken down.
Automated technology for drones has improved substantially in recent years because of better graphics processing units and a culture of open source code sharing. Still, the biggest developments in drone automation have been confined to the lab. The automated drones programmed for the racing series, flying at speeds approaching 70 mph, are unlike anything the general public has seen.
“The things that have been that fast in the lab don’t go that fast in the real world,” says Chelsea Sabo, the technology lead of the contest and a software engineer at Lockheed Martin. “This is the first time that we’ve really taken a lot of this out of the lab and put it in a realistic environment and seen what it can do.”
Lockheed Martin also wants to get the average person more excited about drones. Americans have a NIMBY-like relationship with the flying devices. According to Pew, the most common reaction for an American who spots a drone is curiosity, ranking ahead of nervousness, anger or fear. But a majority of Americans surveyed by Pew said they didn’t want the flying devices near their homes, which isn’t welcome news to companies that envision a future with thousands of drones patrolling the skies and making deliveries.
The drones of the Drone Racing League are smarter, sleeker and faster than any machine the average person can buy.. Nick Horbaczewski started the league in 2015 after attending an amateur drone race behind a Long Island Home Depot. At league competitions, professional pilots steer the drones, glowing in LED light, in environments that look like video games brought to life. Horbaczewski has always envisioned the future of the DRL to feature machines competing alongside human pilots. “There’s lots of people in the world working on autonomous drones,” he says. “What we’ve chosen to do is … take the arena of sports and use it as a crucible to accelerate the development of technology.”
For the AIRR circuit, nine teams were chosen out of nearly 430 applicants earlier this year, and six teams qualified for the finals on Friday night in Austin; it was their fourth competition. The drones are called RacerAIs. They weigh about 7 pounds and have no radar or GPS; they are essentially a pair of robotic eyes, equipped with four cameras that give them a larger field of view than a human. The teams all use the same hardware, so the competition, which consists of five heats, is a test of strategy and code. The drones must be programmed to perceive where they are and where they need to go. The code is set before the race, and the drones make decisions in the race based on the code they have been programmed to run. Most teams start with code designed to propel the drone conservatively through the course at slow speed and use more aggressive codes in the later heats.
In the first contest, in October, the automated drones barely made it off the starting perches before crashing. “A lot of the flights were four seconds,” says MAVLab’s De Wagter, “and you had to learn from that.”
Speed is not the biggest problem for an automated drone. Kocher says the automated drones nearly reached the same top speed as his drone Friday night—though many then crashed. Compared with a piloted drone, an automated drone has the potential to accelerate more quickly based on reaction times. The major challenge is programming a drone to recognize an obstacle and steer through or around it. And the faster the drone goes, the harder it is for programmers to make the adjustments that allow the drone to make the correct decision.
“You basically need to anticipate,” says Kocher, who has a PhD in physics. “I have a mental representation in how I can ride this fine line. But for them they need to write the physics model that knows already what’s going to happen and know in advance how it’s going to work out.”
Subtle changes to the visual or auditory environment that mean nothing to a human can confuse drones and other devices dependent on machine learning. On the Austin course, for instance, the gates featured logos of checkered flags that required MAVLab to reconfigure its code. “The human is not distracted by the flags,” De Croon says.
The MAVLab teammates have been working with autonomous drones for about a decade and racing drones for the last three years. Most recently, they developed what is being hailed as the world’s smallest autonomous racing drone. Building on that experience, the team found the RacerAI drone worked most efficiently using only one of its four cameras.\
“It’s better to have lower quality, high frequency (processing) than good, slow solutions, especially when you start racing and everything gets to be a blur and the lighting conditions vary from one setting to the other,” De Wagter says.
MAVLab and Kocher agree that on simpler courses like those in Austin the automated drones may prevail within the next few years. For the complicated courses used regularly by DRL, it will take longer for automation to catch up.
In other man versus machine contests, like chess, Kocher says, the machines can “brute force” the human out of the contest by calculating far more moves ahead than a person. The unpredictability of drone racing will make it more difficult for the machine.
“Drone racing,” Kocher says, “is a game where they cannot brute force me.”